Machine learning: what are its limitation and potential?
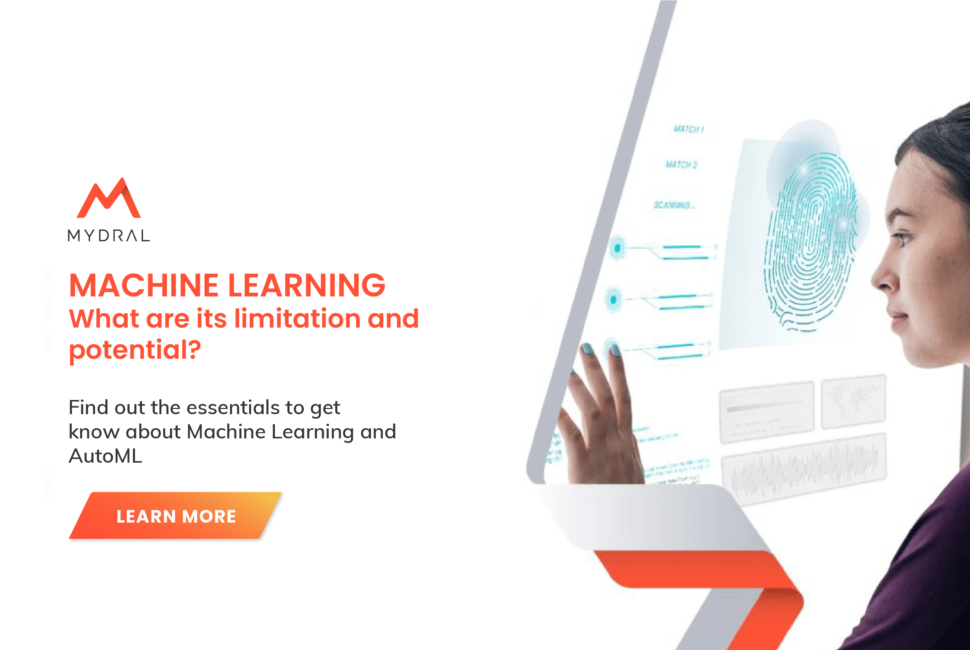
Machine Learning makes data apprenticeship easier. An algorithm transmits predictions following analyses.
What does machine learning signify and how does it work?
Machine learning allows the automatic apprenticeship of a device based on data. We use a mathematics and statistics approach. It brings up to predictions depending on the client’s needs.
By the way, after having to deal repeatedly with data, formulas refine themselves. In the same way, we are aware that before any data’s importation, it is necessary to clean and to prepare it so it can guaranty a good analyze.
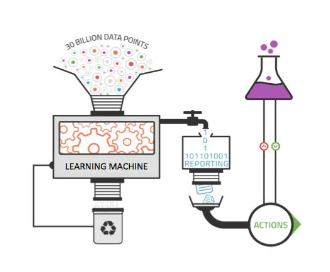
Thus, the machine finds the patterns, understands, and improve its performances in the execution of a specific task, even without being explicitly programmed to do it in order to offer the best fitted solution.
For the apprenticeship through a machine, Mydral uses the solution KNIME.
The essential terms to remember:
- Dataset
A collection of related sets of information that is composed of separate elements but can be manipulated as a unit by a computer.
It often appears as a dashboard or a data matrix.
- Feature
Variables of a dataset that we use to predict what we are looking for.
- Target
Variable of the dataset that we would like to predict, the one that must be explained by our model.
- Outliers
Data that is especially high or low compared to the values’ majority we observe for a variable. Thus, they are either extreme (but possible) « I am 90 years old », either aberrant (so impossible) « I am 180 years old ».
The machine learning usedness
Thanks to the crossing between data and algorithm, it is possible to establish tendances. It allows the client to make a decision more than an other and improve some performances.
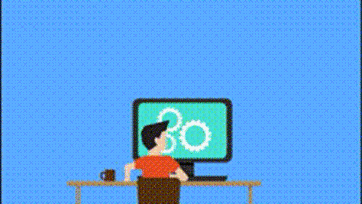
Moreover, it is essential since it allows to report on different reports and constitutes a good complement to a summer up since its predictions can catch the intention after the reading off a report under the format of a dashboarding or of a story telling.
However, machine learning does have limits. To learn, models are in need of a large amount of data. Some models can’t be perfectly analyzed. It is necessary to regularly analyze the performance indicators. Thus, a verification is important to avoid interpretations’ mistakes and the algorithm choices.
The transition to the automated ML
KNIME developed the approach of an integrated and continuous deployment. However, if we would add one case or even modify what we try to predict, we would end up reconfiguring the settings of a single node all along the formatting working flux. Which is why can be integrating the atomized apprenticeship solution (AutoML), for the formation and the deployment of production workflows for the classification of the automatic apprenticeship. Despite their popularity, AutoML solutions can take a lot of time to establish and are efficient only for a subset of automatic apprenticeship problems.
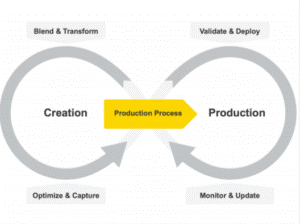
Moreover, the data scientist remains important because the Automated ML only joins as an assistant. In short, we can not delegate everything to the machine. It only simplifies the steps (data treatment, characteristics engineering, extraction of the characteristics and method of their selection) that an expert programs.
To go any further!
You wish to see your last article on our partner KNIME? In reasons of multiple functionalities and its interactions, Twitter is a mine of information. Find out how to analyze behaviors on Twitter and to measure feelings on your brand or your products with an API KNIME.
Check out the article HERE.
Author Profile
Latest entries
News17 August 2022« Data driven marketing », what does it stand for?
News25 July 2022[News] Tableau and Salesforce: CRM Analytics
News18 July 2022Data Design – Make your data stunning
How To11 July 2022Combine several Excel sheets on Knime